Primer on AI Chips and AI Governance
Summary
- If governments could regulate the large-scale use of “AI chips,” that would likely enable governments to govern frontier AI development—to decide who does it and under what rules.
- In this article, we will use the term “AI chips” to refer to cutting-edge, AI-specialized computer chips (such as NVIDIA’s A100 and H100 or Google’s TPUv4).
- Frontier AI models like GPT-4 are already trained using tens of thousands of AI chips, and trends suggest that more advanced AI will require even more computing power.
- Governments can likely regulate the large-scale use of AI chips.
- It would be hard to enforce regulations on things like data or algorithms, because it is very easy to copy, transmit, or store them. In contrast, AI chips are physical in nature, so governments can more easily track and restrict access to AI chips. As a result, regulation of AI chips is relatively feasible.
- AI chips are manufactured through an extremely complex, global supply chain, in which a small number of companies and countries dominate key steps. As a result, coalitions of only a few actors could require other actors to meet safety standards in order to import AI chips. Any state would likely find it extremely difficult to manufacture AI chips on their own.
- Since AI chips are so specialized, they can be regulated without regulating the large majority of computer chips.
- Because of the above, governments can likely govern frontier AI development by regulating AI chips. However, governance of large numbers of AI chips faces large potential limitations:
- Due to hardware progress and algorithmic advances, the number and sophistication of chips needed to train an AI model of a given capability decreases every year. As a result, regulation of AI chips can only temporarily regulate the development of potentially dangerous AI models. However, even temporary measures may buy time that is crucial for safety.
- Well-resourced actors may be able to support frontier AI development without relying on cutting-edge AI chips, by spending substantially more. If so, then strategies that rely on excluding these states from frontier AI development may be infeasible.
- The compute supply chain may become more distributed in the future (e.g. due to new hardware paradigms), making it difficult to enforce restrictions.
- Governments have already taken major actions to regulate AI chips: the US and allies have restricted the export of AI chips (and the equipment needed to produce them) to China.
- Regulation of AI chips could also promote international cooperation on AI safety, such as by enabling verification of international agreements on AI development.
- Aside from regulating AI chips, it may be feasible and effective to regulate other specialized hardware used for frontier AI development, such as networking equipment in data centers; there has been very little research on this.
(The high-level structure of this article draws from part of a forthcoming paper by Brundage et al.: "Computing Power and the Governance of Artificial Intelligence.")
Frontier AI development uses tens of thousands of AI chips.
Many technologies, such as cars, are largely regulated at the point of use. However, particularly dangerous technologies such as nuclear reactors or chemical agents require restrictions not only of the final product, but also of various components used to produce them. Similarly, due to the potentially catastrophic scale of harm from advanced AI models, safeguarding these models may require governing their ingredients.
AI-specialized computer chips matter for AI policy because they are likely a crucial and governable ingredient of frontier AI development. We will begin by discussing the first part of that: the crucial role these chips play for frontier AI development, now and in the future.
To train a frontier AI model, computing devices need to do vast numbers of operations (such as multiplying numbers). For example, GPT-3 (a precursor of ChatGPT) was trained with approximately 3e23 (that's 300,000,000,000,000,000,000,000) computer operations.
As will be familiar to some readers, the devices that do computer operations are called computer chips. There are computer chips in everything from laptops to microwaves, but the chips used for cutting-edge AI development are highly specialized chips, and they are operated in large numbers within specialized buildings known as data centers.
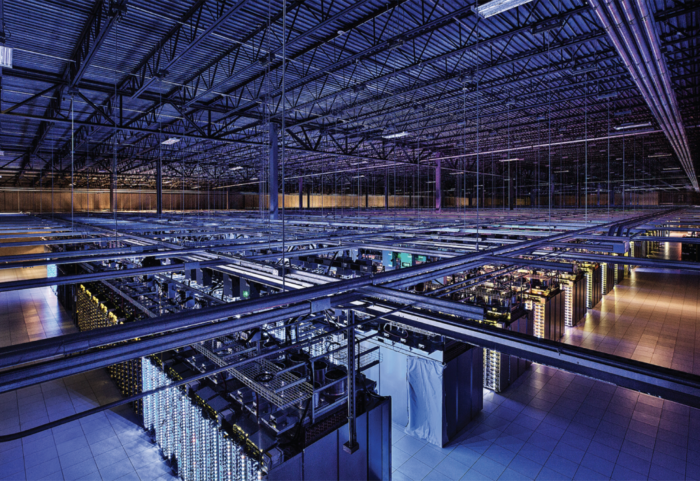
A large Google data center.
Some chips ("AI chips") are vastly more efficient than others, for AI development. A report from Georgetown's Center for Security and Emerging Technology explains:
Training a leading AI algorithm can require a month of computing time and cost $100 million. This enormous computational power is delivered by computer chips that not only pack the maximum number of transistors—basic computational devices that can be switched between on (1) and off (0) states—but also are tailor-made to efficiently perform specific calculations required by AI systems. Such leading-edge, specialized “AI chips” are essential for cost-effectively implementing AI at scale; trying to deliver the same AI application using older AI chips or general-purpose chips can cost tens to thousands of times more.
In recent years, frontier AI development has been done with up to tens of thousands of AI chips. Without access to large numbers of these chips, such AI training would take several years to decades to complete. Alternatively, less advanced chips could be used in much greater quantities.
Some further context on AI chips:
- As of 2023, some examples of (near-)cutting-edge AI chips are A100s, TPUs, and H100s. Most cutting-edge AI chips are specialized versions of a broader category of AI chips, called GPUs, and AI-specialized chips more broadly are called AI accelerators.
- Computer chips have been rapidly becoming more efficient for decades. Researchers at Epoch AI estimate that, in recent decades, the number of GPU operations that a dollar can buy has doubled every ~2.5 years.
- Another term for computing operations is "compute." Relatedly, the research and policy subfield focused on governing AI chips is often called “compute governance.”
- Training an AI model from scratch requires much more compute than running one copy of it after it has been trained.
- However, providing an AI service to millions of users requires running many copies of an AI model. The computational costs of this can easily surpass those of training the model.
- A trained AI can be “fine-tuned”—trained a little further. Fine-tuning might be done to change the format or style of an AI model’s output, to teach the AI a new skill, or to specialize the AI for a particular task. Fine-tuning typically requires significant compute: much less than the initial training (“pre-training”), but more than running one copy of it.
Frontier AI development already requires much computing power; next, we will examine why this trend is likely to become even more extreme.
Trends suggest that more advanced AI will be trained with even more compute
When an AI model is trained with more compute, it tends to have more advanced capabilities. For example, an AI model trained with more compute tends to be more successful at predicting the next word in a sentence, or better at achieving high scores in games. These trends are so consistent that they have been used to make verified predictions, and they can be described mathematically by what are known as "scaling laws." (However, it is unclear how far “scaling laws” will generalize, and their numerical predictions can be difficult to interpret qualitatively.) A visual example of a scaling trend is the following graph, from OpenAI, which shows prediction errors decreasing as compute increases:
The importance of computing power for AI capabilities is reflected in the modern history of AI. Epoch AI estimates that, since 2010, the amount of compute used to train milestone AI systems has grown by ~4x each year. AI developers have achieved this growth by using increasingly specialized chips, in growing numbers, for longer periods of time. Increased compute allows developers to train more complex AI models on more training examples. Growing compute use has come with rising costs, which have led frontier AI development to shift from being dominated by academia to being dominated by industry.
A prominent hypothesis is that very advanced AI capabilities will not require major breakthroughs in algorithms; instead, the main bottleneck is scaling compute. While this may be unintuitive, many qualitatively new AI capabilities have been achieved in recent years primarily by scaling up existing AI designs, rather than with profound research insights.
We have seen that large numbers of AI chips are crucial for frontier AI development, and that future frontier AI development will likely rely on even more AI chips that are even more specialized. This implies that, if governments could regulate how large numbers of AI chips are used, that would enable them to regulate frontier AI development. As we will see next, governments can in fact (probably) regulate how large numbers of AI chips are used, enabling regulation of frontier AI development.
Governments can likely regulate how large quantities of AI chips are used.
Inherent properties of chips facilitate regulation. It would be hard to enforce regulations on things like data or algorithms; it is challenging to monitor their proliferation, as they can be easily distributed. In contrast, AI chips are physical objects, rather than information, so it is more feasible for governments to monitor and regulate their distribution.
Beyond inherent properties of chips, the AI chip supply chain further facilitates regulation, because key steps in the global supply chain are dominated by a few countries (especially the US and its allies). Additionally, no actor could easily stop relying on this global supply chain; analysts have found that it would be “incredibly difficult and costly for any country” to manufacture AI chips on its own—even the United States or China. As a result, some countries have outsized leverage over how AI is developed globally; they can set conditions for technology exports.
To see just how concentrated the AI chip supply chain is, consider the following (simplified) overview of the AI chip supply chain, and the roles different countries play. The following overview is based on a report from Georgetown’s Center for Security and Emerging Technology.
- Designing AI chips.
- US companies dominate this market. Today’s frontier models are exclusively trained on chips designed by NVIDIA and Google, both US companies.
- Making the machines that manufacture AI chips (“semiconductor manufacturing equipment”).
- All the most advanced equipment for one key manufacturing step is produced by a single company in the Netherlands, ASML. (This equipment is known as “EUV lithography” equipment.) This monopoly is due to the extreme technical and logistical complexity of ASML’s work. Aside from lithography, the Netherlands, US, and Japan have large roles in other semiconductor manufacturing equipment.
- Fabrication of AI chips.
- As summarized in a Forbes article, “There are only three companies in the world that can build advanced chips anywhere near the leading edge of today’s most advanced semiconductor technology: TSMC [a Taiwanese company], Samsung [a South Korean company] and Intel [a US company]. Of these three, only one [TSMC] can reliably build the most advanced chips, including chips like Nvidia’s H100 GPUs that will power the next generation of artificial intelligence.” [Bolding added.]
- Assembling AI chips.
- After core components have been fabricated, they are assembled into a finished chip, tested, and packaged. Previous analyses have not identified this step as a supply chain chokepoint, instead finding that it is often outsourced and China has a significant minority of the market share. Still, some packaging technology has reportedly caused supply chain bottlenecks amidst rising chip demand.
- Selling access to AI chips over the Internet (“cloud compute providers”).
- US companies lead, with three US companies—Amazon Web Services, Microsoft Azure, and Google Cloud—holding a combined ~65% market share.
The report from the Center for Security and Emerging Technology also provides a summary table (for our purposes, no need to worry about the details).
Here are some further details that illustrate the sophistication and expenses of the AI chip supply chain, and the difficulties a country would face in manufacturing AI chips on its own:
- “The half-trillion-dollar semiconductor supply chain is one of the world’s most complex. The production of a single computer chip often requires more than 1,000 steps passing through international borders 70 or more times before reaching an end customer” (Khan et al., 2021).
- As one step in ASML’s machines, microscopic drops of tin are vaporized as they fall, by being struck by a laser 50,000 times a second.
- “For a further sense of scale, China’s national-level chip subsidies of $18.8 billion by 2018 are dwarfed by just TSMC’s $34 billion investment on new fabs. The outsized scale of the chip industry challenges even China’s appetite for industrial subsidies” (Khan and Flynn, 2020).
- “Given the complexity and expense of fabricating state-of-the-art chips, only a few chip fabrication factories (“fabs”) can profitably operate at or near the state-of-the-art. [...] State-of-the-art chip fabs now cost more than $10 billion to build, making them the most expensive factories ever built” (Khan and Flynn, 2020).
We have seen evidence for two premises. Previously, we saw that large numbers of AI chips will likely continue to be crucial for AI development. Here, we have seen that large numbers of AI chips are likely governable. Together, these premises suggest that governments can likely govern frontier AI development by governing AI chips. (This talk introduces some potential options for governing AI chips.) Next, we will consider potential limitations of compute governance
Three potential limitations of compute governance
Even if there were the political will for relevant governments to implement ambitious forms of compute governance, there are several ways these efforts might fail to achieve their goals:
- Small amounts of compute may pose severe security threats.
- For a given AI capability, engineers tend to find ways to achieve it with less and less compute over time. This trend is “algorithmic progress.” Epoch AI estimates that the compute requirements for AI models to achieve a given accuracy in recognizing images has fallen by a factor of ~2.5 per year since 2015. (There has been little public work to quantify similar trends in other domains.)
- If the above trends continue (as we might expect, given ongoing innovation and incentives to reduce compute costs), then it will eventually be feasible to train AI models with dangerous capabilities, without relying on cutting-edge chips.
- Additionally, as high-end hardware becomes cheaper, it tends to become more widely used (e.g. in personal computers), making it logistically and politically harder to regulate it.
- As a result, it will likely become harder each year to regulate AI models of any given capability level.
- Despite this, frontier AI regulation could still be valuable; frontier AI regulation could make it possible to prepare for the proliferation of advanced AI capabilities. For example, before AI models with advanced hacking abilities are widely accessible, the same AI models could be used to strengthen defenses against hackers. More generally, regulation could ensure that the first use of frontier AI systems is to develop defensive technologies, evidence about risk, and safe training methods, in preparation for later proliferation. However, it is unclear whether safety work could be done quickly enough—before dangerous AI models have proliferated.
- (This issue is discussed further in this podcast interview.)
- Well-resourced states may carry on with advanced AI development without cutting-edge, AI-specialized chips.
- Well-resourced states could buy large numbers of older-generation AI-specialized chips and use them to train advanced AI models, despite not having access to more efficient, newer hardware.
- As a result, strategies that rely on excluding well-resourced states from advanced AI development might be infeasible. Compute governance strategies that do not rely on that may still work.
- Drastic changes in AI chip manufacturing may make export restrictions infeasible.
- There is some work aimed at replacing the currently dominant approach to building AI chips with very different approaches, such as brain-inspired hardware (neuromorphic computing) or hardware that uses light instead of electrons (optical computing). However, these approaches face uphill battles in competing with the immense amount of innovation that has gone into the current AI chip supply chain. Still, the success of an alternative hardware paradigm might mean that a small amount of hardware, or hardware that many countries can develop on their own, would suffice for dangerous AI development.
Governments have already taken major actions on AI chips: restricting their export to China.
From 2020 to 2023, the US and its allies have placed mounting restrictions on exporting AI chips (and the machines that make them) to China. This history is relevant both because of its impact on AI, and also as an example of how the US (with allies, as well as unilaterally) can exercise international influence on AI.
- In 2020, the Dutch government, reportedly under US pressure, declined to renew ASML’s license to export its most advanced chip-making machines to China.
- In 2022, the US government unilaterally established sweeping restrictions on exporting AI chip technology to China. In establishing these restrictions, the US asserted authority over some non-US-based companies, on the grounds that they were using US-made software and equipment or US employees.
- While US export controls on AI chips were initially unilateral, multiple US allies with key roles in the AI chip supply chain—namely the Netherlands and Japan—have since imposed related export controls. Additionally, Germany is reportedly in talks to impose its own controls on relevant exports to China. A report from CSIS argues that export controls from allies are crucial for the effectiveness of US export controls; otherwise, some technologies that the US refused to export to China would simply be provided by non-US suppliers.
More broadly, compute governance can slow the proliferation of the ability to develop frontier AI or deploy it at mass scale, ideally limiting the access of malicious or reckless actors.
Compute governance could enable international cooperation
Though AI chip policy so far has largely occurred in the adversarial context of export controls, that is not the only possibility for governing AI chips. AI chips might also be used to verify compliance with international agreements on AI, or to otherwise advance cooperation. For example, mechanisms built onto AI hardware may be able to verify and enforce compliance with AI regulations or agreements, in privacy-preserving ways. These possibilities are introduced with more detail in two other pieces that are also core readings in this course (as of August 2023):
- Introduction to Compute Governance (Heim, 2023)
- What Does it Take to Catch a Chinchilla? (Shavit, 2023)
As a third potential application of AI governance—in addition to restricting access and facilitating cooperation—compute subsidies can promote beneficial AI projects, such as safety research.
Regulation of data centers or networking equipment might be promising
So far, we have focused on how AI chips might enable AI governance. Still, it may also be useful to regulate non-chip parts of data centers (large facilities where many chips are operated). For example, perhaps owners of AI-relevant data centers could be required to verify users’ compliance with AI regulations, by using mechanisms built onto networking equipment (equipment that coordinates the use of multiple chips). As of August 2023, there has been minimal research into the technical feasibility of this.
There are potential advantages to regulating AI data centers or networking equipment without regulating AI chips. Doing so may turn out to be more technically feasible, and it may be politically easier than overseeing all AI chips. One factor is that the AI data center market is increasingly centralized; more AI companies are remotely accessing data centers owned by a few major tech companies (“cloud compute”). However, the effectiveness of such regulations is an open question. On one hand, assuming technical feasibility, data center regulations could raise the costs of dangerous AI development, by requiring violators in regulating countries to secretly build data centers. Regulations may also have international effects, if there are governable “choke points” in the supply chain for data centers’ equipment (beyond chips). Still, it is unclear whether these costs would exceed incentives to violate regulations.